If we are going to reduce the carbon footprint of data centers we need to use computing resources more efficiently. If processes always made use of data center facilities in a regular way, it would be an easy game. However, the resources of a data center are used by customers (more often customers of customers) in ways that are not only unpredictable, but lacking in transparency - even in real time. Data center staff are not permitted to observe the processes being used by their customers. So how do you allocate resources efficiently, when you are being handed black boxes?
To solve a complex question like this, it would be appear that a crystal ball is necessary. The crystal ball being developed at EPFL is called CloudProphet.
Data center resources are used by customers in ways that are not only unpredictable, but also lacking in transparency, as data center staff are not permitted to observe the processes being used by their customers. To solve the problem of how to allocate computing resources efficiently, it would appear that a crystal ball is necessary. The crystal ball being developed at EPFL is called CloudProphet.
"Companies like Google and Amazon provide virtual machines for customers," explains David Atienza, head of the Embedded Systems Lab in the School of Engineering. "But these customers do not tell you anything about what they are actually doing, and we are not permitted to look inside. Therefore, the behavior of applications is hard to predict – they are black boxes."
By identifying application processes from the outside, and basing performance prediction only on hardware counter information, CloudProphet learns to anticipate an application's demands on resources. Neural networks multiply in a balletic form of machine learning, building up a picture of the predicted requirements.
"Data centers do have diagnostic tools for the identification and performance prediction of applications, but they can only claim an improvement rate of 18%. We are achieving results that are orders of magnitude above that," explains ESL PhD student Darong Huang. "Results that have just been published in IEEE Transactions on Sustainable Computing. We hope that CloudProphet will pave the way to a more intelligent resource management system for modern data centers, thus reducing their carbon footprint."
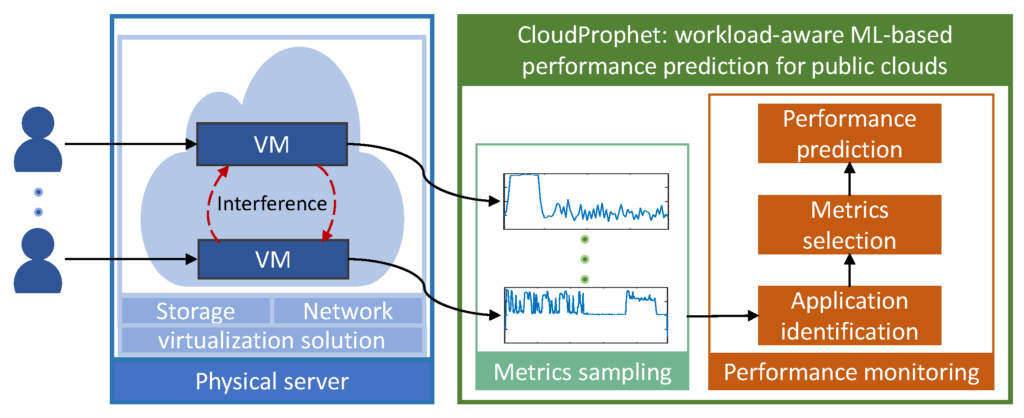
Atienza explains that PhD students and postdoctoral fellows are funded by industry to work on these projects, maintaining systems at a distance.
"Industry project managers are invited to give advice on the physical and logistical constraints in place so that we get as close as possible to a real-world application,” he says.
The new EPFL data center, the CCT building (Centrale de Chauffe par Thermopompe), presents another opportunity to apply this technology to the real world. In collaboration with Mario Paolone's Distributed Electrical Systems Lab, the EcoCloud Center, and the EPFL Energy Center, a framework will be set up to put these systems to the test.
"Once we can see up to what extent the carbon data footprint is reduced, we can look at whether the next step is to license the software, or to start a spin-off company,” concludes Atienza.
All this is in the future, and nobody can predict the future. Although with the benefits of machine learning, collaboration between research teams and dialogues with industry, we can get close…
Publication:
https://infoscience.epfl.ch/record/307296?&ln=fr
https://ieeexplore.ieee.org/document/10415550
Project page:
Text: John Maxwell
Photo: Alex Widerski