Dr. Gourav Datta of University of Southern California Viterbi, Electrical Engineering, will give a presentation on the
1st June at 12 o'clock
in lecture theatre BC420, followed by a pizza lunch.
In-Sensor & Neuromorphic Computing are all you need for Energy-Efficient Deep Learning
This talk will focus on two research thrusts in energy and latency-efficient edge intelligence.
The first thrust is inspired by the fact that spiking neural networks (SNNs) are a promising low-power alternative to compute and memory-expensive DNNs, due to their high activation sparsity and use of accumulates (AC) instead of expensive multiply-and-accumulates (MAC). However, most existing SNNs require multiple time steps for acceptable inference accuracy, hindering real-time deployment and increasing energy consumption. This motivates the need for novel training frameworks to optimize source DL models, their conversion to target SNNs, and subsequent SNN fine-tuning, which I will discuss in this talk.
The second thrust is inspired by the fact that modern image sensors generate huge amounts of high-resolution visual data that typically must be transferred to downstream CPUs/accelerators for computer vision (CV) processing. This data transfer requires significant bandwidth and can dominate the total energy consumption, particularly when the CV processing is heavily optimized using SNNs.
I will discuss a novel in-sensor computing paradigm based on CNNs, coupled with intelligent algorithm-hardware co-design that can mitigate this concern. This paradigm is the first to demonstrate the feasibility of enabling all the computational aspects of modern CNN layers inside image sensors. Coupled with the optimized SNNs, this paradigm can reduce the total system energy-delay product (EDP) of several on-device CV workloads by two orders of magnitude compared to existing approaches without significantly affecting the performance.
Gourav Datta
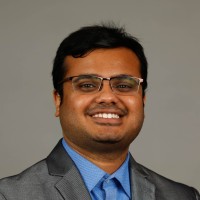
Dr. Gourav Datta (Graduate Student Member, IEEE) received the B.Tech. Degree in instrumentation engineering with a minor in electronics and electrical communication engineering from the Indian Institute of Technology, Kharagpur, in 2018. He has just completed a PhD degree at the University of Southern California, USA. His research interests include energy and latency-efficient algorithm-hardware co-design for machine learning at the edge. He is a finalist of the Qualcomm Innovation fellowship 2022 (North America), a recipient of the IEEE Graduate Fellowship on Applied Superconductivity, and a Ming Hsieh Ph.D. scholar. During his PhD tenure he has published 20 peer-reviewed papers in top-tier venues such as Scientific Reports, Frontiers in Neuroscience, TCAS-I, DATE, and WACV, among others.