Demetri Psaltis and the return of optical neural networks
It is not often that professors from EcoCloud are featured in the pages of The Economist. In an article from December 2022, entitled “Artificial intelligence and the rise of optical computing”, the author had this to say:
“The idea of turning neural networks optical is not new. It goes back to the 1990s. But only now has the technology to make it commercially viable come into existence. One of the people who has observed this transition is Demetri Psaltis, an electrical engineer then at the California Institute of Technology (Caltech) and now at the Swiss Federal Institute of Technology in Lausanne. He was among the first to use optical neural networks for face recognition.” [1]
Although Demetri Psaltis was indeed among the first to research optical neural networks, even before the 1990s, many of his most recent publications deal with this same topic. This includes one from earlier this year – an article in Nanophotonics, [2] which was already cited by another author in the same journal within a month. [3] However, that does not mean that Prof. Psaltis has been focused solely on this subject for the last forty years: it is a lot more complicated than that, and a lot more interesting.
The dawn of optical neural networks
Neural networks are a technology that gather data in a way inspired by our nervous system – a lattice of facts (nodes) and connectors (edges). They use artificial intelligence to increase the depth of their own knowledge, for the benefit of the user. According to ChatGPT (which is itself a neural network), the largest neural network in the world is Google’s GShard, with over a trillion parameters. This is a far cry from Prof. Psaltis’ ground-breaking work on optical neural networks in the 1980s:
“Our first neural network had thirty-two nodes. Not much, but it was a start. It is not a coincidence that the rise of neural networks happened just as the home computer revolution was taking off!”
ChatGPT is a neural network, which uses machine learning to gather information on a range of subjects, increasing its knowledge autonomously, and using natural language processing to provide answers to users’ questions.
Wikipedia is a not a neural network, because it is maintained by human volunteers, who provide, edit and correct the information stored in its databases. It is much more reliable than ChatGPT – for the moment, at least …
The optical neural networks revolution had barely begun, but – as pointed out in the Economist – Psaltis was one of the pioneers. At the bridge between the domains of physics and computing, this ground-breaking concept involves harnessing light, and using it to create neural networks. Optical computers transport data with photons rather than electrons, offering the possibility to make use of the amazing properties of light – its parallelism and its speed – as a form of parallel processing. This not only results in fast data processing, but runs with lower power consumption than a traditional computer.
In an article published in Applied Optics in 1985, Psaltis described the basis of this new technology:
“Optical techniques offer an effective means for the implementation of programmable global interconnections of very large numbers of identical parallel logic elements. In addition, emerging optical technologies such as 2D spatial light modulators, optical bistability, and thin-film optical amplifiers appear to be very well suited for performing the thresholding operation that is necessary for the implementation of the model.” [4]
Implementing this model was a breath-taking challenge, and one of many that made the reputation of Demetri Psaltis, who was then a researcher at Caltech. In order to get an idea of how important his research was to the development of optical computing, one only has to look up the phrase Optical neural network in Wikipedia. The first reference in the corresponding article is to a 1988 paper by D. Psaltis, [5] the title of which – Adaptive Optical Networks Using Photorefractive Crystals – conjures up images of physicists wrangling beams of light, like frontiersmen taming wild horses.
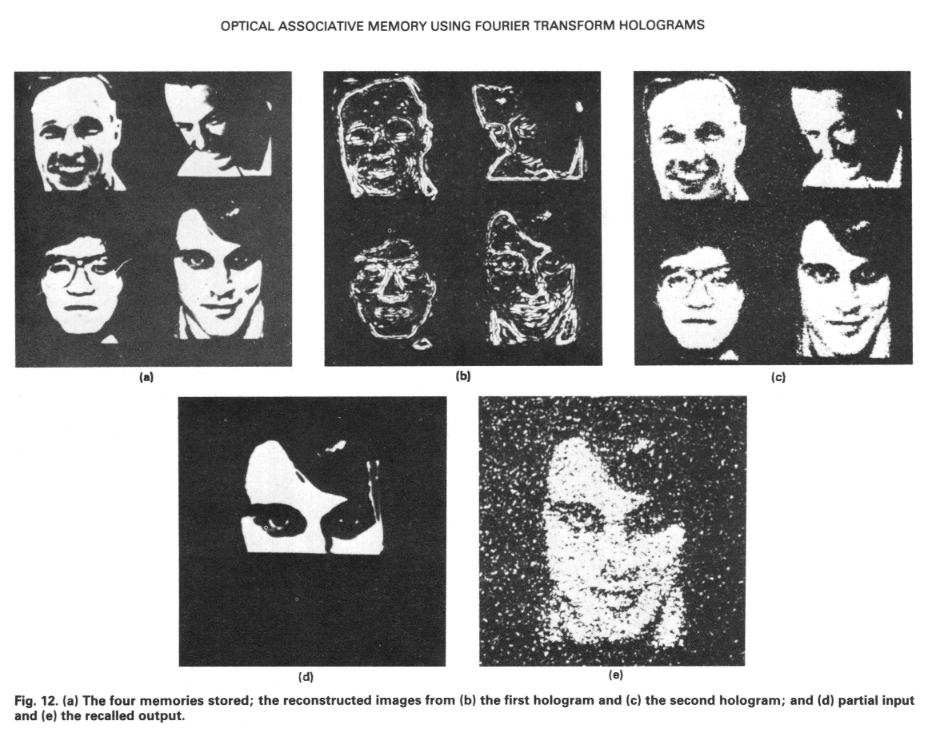
There was, however, a problem: “Back then my thing was building optical computers. We built them then, and we’re building them now. The difference is that back then we needed enormous databases, massive memory, super-fast networks. We didn’t have any of that.”
The secret is in diversity
According to an article published in 2021, in APL Photonics: “The pioneer himself, Psaltis, declared in the 1990s that he was abandoning optical neuromorphic computing for two reasons:
- lack of practical devices that can be integrated and
- insufficient knowledge of complex neural networks.” [7]
Chemical advances in optical components, reconfigurable optical circuits, low-energy applications for optical devices – all of these concepts feature in the work Prof. Psaltis carried out at Caltech up until 2007, and at EPFL thereafter. Another dominant theme is microfluidics. In 2006 he was working on “devices in which optics and fluidics are used synergistically to synthesize novel functionalities. Fluidic replacement or modification leads to reconfigurable optical systems, whereas the implementation of optics through the microfluidic toolkit gives highly compact and integrated devices.” [8]
After a long absence, however, neural networks made a return. Perusing his list of publications, the phrase “neural networks” is not to be found from 2003 until 2019. Then it is back – with a vengeance.
The return of the neural network
In 2016, Prof. Psaltis wrote the following in an article entitled “Optical Computing: Past and Future”:
“All-optical information processing has a checkered past — but technological developments, tougher problems and the rise of big data are all prompting a new look.” [9]
Psaltis confirms that changes to the landscape have occurred: “What we have now is Google, supercomputers, fibre optic networks. So everybody is talking about optical neural networks again. In industry, over a billion dollars have been invested in this technology!”
During the course of this interview, doctoral students would occasionally drop in to the office of Prof. Psaltis, and his enthusiasm for their discoveries is immediately visible and audible. So what work are he and his researchers doing now, and how do neural networks feature in it? We can look briefly at two examples.
Using optics to build neural networks
The neural networks of the 1980s have given way to the much larger Deep Neural Networks (DNN) of the modern era, but there is a certain amount of continuity in what Prof. Psaltis was pioneering then, and what he is aiming to achieve now.
One invention in particular – Multimode Fibers (MMF) – has been a game changer. In a paper in Nanophotonics (2022), written in collaboration with Prof. Christophe Moser of the Laboratory of Applied Photonics Devices (LAPD), Psaltis wrote: “In what follows, we review recent works that use modern data-driven deep neural networks (DNNs)-based methods for imaging, projection in scattering media and specifically MMFs. We show that modern data-driven deep neural networks considerably simplify the measurement system and experiments and show that they can correct external perturbations as well. We also show recent works that MMF can be used as a medium to do optical computing.” [10]
Multimode Fibres uses multiple rays of light simultaneously, with each ray of light running at a different reflection angle. They can therefore be used to transmit masses of data over the short distance between one part of a computing processor and another. Putting MMF to work in building Deep Neural Networks means going really big, really fast – with lower energy use than traditional binary technology.
At this point, the professor turns things around: as well as using optical computing to build deep neural networks, he is building neural networks that can help design optical components.
Using neural networks to build optics
As neural networks grow in depth and speed, they are being utilised to produce work that only humans could previously do. Dall-E 2 is used to generate images, ChatGPT can churn out essays – but the work is arguably poor quality compared to that of a true professional. MaxwellNet is different.
“We built a deep neural network out of Maxwell’s equations. With MaxwellNet the laws of physics are the rules. So you build your model, and if these rules are not met there will be an error. You’re using the physics described in Maxwell’s equations in order to test a simulation.”
MaxwellNet was tasked with helping to design lenses, components for optical computers, as described in a paper from last month in APL Photonics: “MaxwellNet maps the relation between the refractive index and scattered field through a convolutional neural network. We introduce here extra fully connected layers to dynamically adjust the convolutional kernels to take into account the intensity-dependent refractive index of the material. Finally, we provide an example of how this network can be used for the topology optimization of microlenses that is robust to perturbations due to self-focusing.” [11]
So here is a deep neural network with a difference, as Prof. Psaltis explains: “You don’t have to compile a database, you don’t need to train the model, so much as to let James Clerk Maxwell decide if your model will work or not.”
It sounds like science fiction, but it is just another chapter in this lifelong, ongoing adventure: harnessing light, surfing photons, conjuring matrices of data and watching them dance.
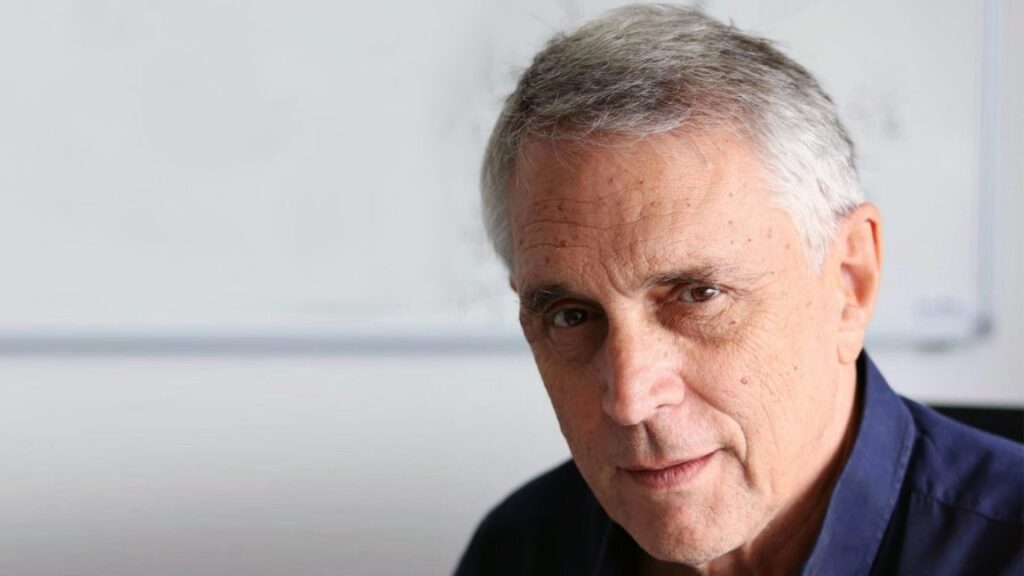
[1] “Artificial Intelligence and the rise of optical computing”, The Economist, 20th December 2022
[2] Dinc, Niyazi Ulas, Saba, Amirhossein, Madrid-Wolff, Jorge, Gigli, Carlo, Boniface, Antoine, Moser, Christophe and Psaltis, Demetri. “From 3D to 2D and back again” Nanophotonics, vol. 12, no. 5, 2023, pp. 777-793. https://doi.org/10.1515/nanoph-2022-0512
[3] Brunner, Daniel, Soriano, Miguel C. and Fan, Shanhui. “Neural network learning with photonics and for photonic circuit design” Nanophotonics, vol. 12, no. 5, 2023, pp. 773-775. https://doi.org/10.1515/nanoph-2023-0123
[4] Nabil H. Farhat, Demetri Psaltis, Aluizio Prata, and Eung Paek, “Optical implementation of the Hopfield model,” Appl. Opt. 24, 1469-1475 (1985)https://opg.optica.org/ao/fulltext.cfm?uri=ao-24-10-1469&id=28380
[5] Demetri Psaltis, David Brady, and Kelvin Wagner, “Adaptive optical networks using photorefractive crystals,” Appl. Opt. 27, 1752-1759 (1988)https://opg.optica.org/ao/fulltext.cfm?uri=ao-27-9-1752&id=31325
[6] Eung Gi Paek and Demetri Psaltis “Optical Associative Memory Using Fourier Transform Holograms,” Optical Engineering 26(5), 265428 (1 May 1987). https://doi.org/10.1117/12.7974093
[7] L. El Sroujia, A. Krishnan, R. Ravichandran, Y. Lee, M. On, X. Xiao, and S. J. Ben Yooa, “Photonic and optoelectronic neuromorphic computing”, APL Photonics 7, 051101 (2022); https://doi.org/10.1063/5.0072090
[8] Psaltis D, Quake SR, Yang C., “Developing optofluidic technology through the fusion of microfluidics and optics”, Nature. 2006 Jul 27;442(7101):381-6. https://www.nature.com/articles/nature05060
[9] Ravi Athale and Demetri Psaltis, “Optical Computing: Past and Future,” Optics & Photonics News 27(6), 32-39 (2016)https://opg.optica.org/opn/abstract.cfm?uri=opn-27-6-32&origin=search
[10] Rahmani, Babak, Oguz, Ilker, Tegin, Ugur, Hsieh, Jih-liang, Psaltis, Demetri and Moser, Christophe. “Learning to image and compute with multimode optical fibers” Nanophotonics, vol. 11, no. 6, 2022, pp. 1071-1082. https://doi.org/10.1515/nanoph-2021-0601
[11] Carlo Gigli, Amirhossein Saba, Ahmed Bassam Ayoub, Demetri Psaltis. “Predicting nonlinear optical scattering with physics-driven neural networks”, APL Photonics 8, 026105 (2023); https://doi.org/10.1063/5.0119186