Energy-constrained and sustainable deep learning
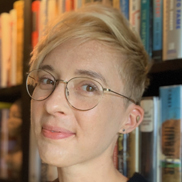
Everyone wants more accuracy, but at what cost?
Making the deep learning community aware of the trade-offs between the performance and carbon footprint of training their models can help guide efficient carbon-aware design. Such efficiency is not just "good for the environment" but can also better guarantee access to predictive analytics in low-resource settings.
This talk addresses some key needs for carbon-aware deep learning, presents a pragmatic tool for doing so, and calls for further collaboration to integrate these approaches into digital Global Health: a massive-data use-case, where efficiency is critical for equitable access to data science.
Mary-Anne Hartley is a clinician and a researcher at EPFL's Machine Learning and Optimization Laboratory.